July 16, 2021
by Andrey Filippov and Fyodor Filippov
Advance Steel 2024: https://abcoemstore.com/product/autodesk-advance-steel-2024/ System Requirements Learn about the system requirements for configuring Advance Steel.
Download links for: video and captions.
This research “Long-Range Thermal 3D Perception in Low Contrast Environments” is funded by NASA contract 80NSSC21C0175
August 4, 2019
by Andrey Filippov
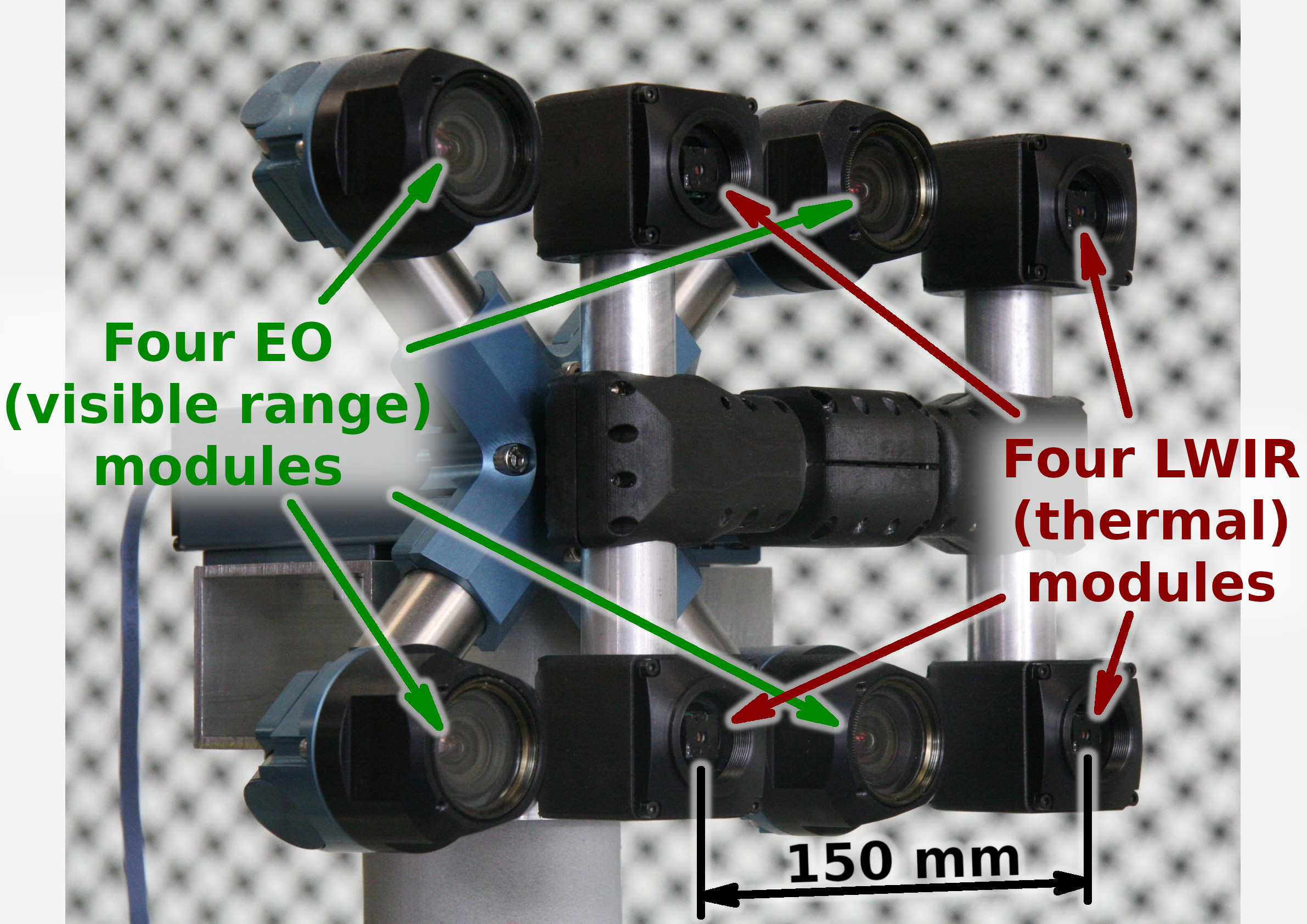
Figure 1. Talon (“instructor/student”) test camera.
Update: arXiv:1911.06975 paper about this project.
Summary
This post concludes the series of 3 publications dedicated to the progress of Elphel five-month project funded by a SBIR contract.
After developing and building the prototype camera shown in Figure 1, constructing the pattern for photogrammetric calibration of the thermal cameras (post1), updating the calibration software and calibrating the camera (post2) we recorded camera image sets and processed them offline to evaluate the result depth maps.
The four of the 5MPix visible range camera modules have over 14 times higher resolution than the Long Wavelength Infrared (LWIR) modules and we used the high resolution depth map as a ground truth for the LWIR modules.
Without machine learning (ML) we received average disparity error of 0.15 pix, trained Deep Neural Network (DNN) reduced the error to 0.077 pix (in both cases errors were calculated after removing 10% outliers, primarily caused by ambiguity on the borders between the foreground and background objects), Table 1 lists this data and provides links to the individual scene results.
For the 160×120 LWIR sensor resolution, 56° horizontal field of view (HFOV) and 150 mm baseline, disparity of one pixel corresponds to 21.4 meters. That means that at 27.8 meters this prototype camera distance error is 10%, proportionally lower for closer ranges. Use of the higher resolution sensors will scale these results – 640×480 and longer baseline of 200 mm (instead of the current 150 mm) will yield 10% accuracy at 150 meters, 56°HFOV.
(more…)
June 18, 2019
by Andrey Filippov
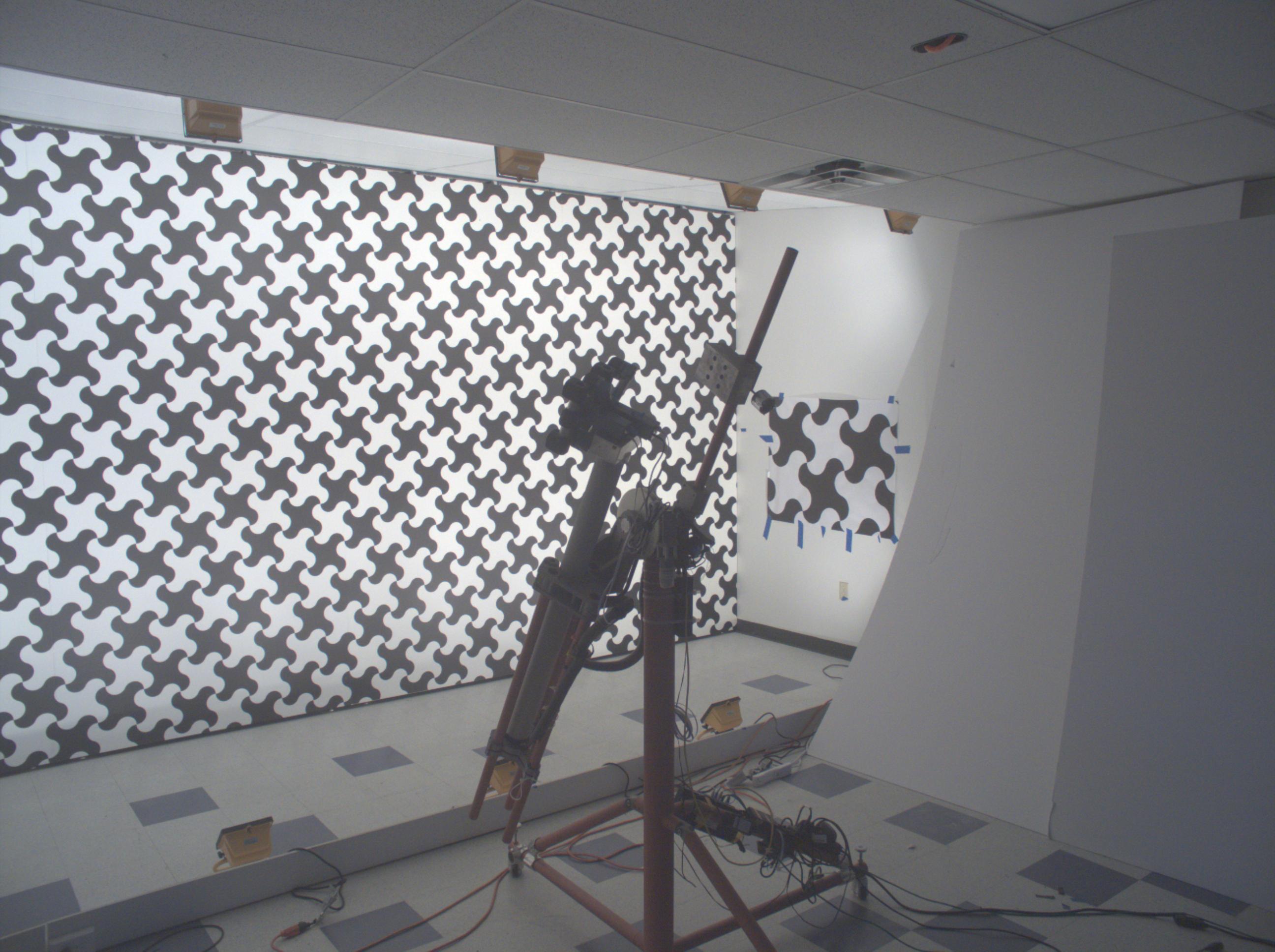
Figure 1. Calibration of the quad visible range + quad LWIR camera.
We’ve got the first results of the photogrammetric calibration of the composite (visible+LWIR) Talon camera described int the previous post and tested the new pattern. In the first experiments with the new software code we’ve got average reprojection error for LWIR of 0.067 pix, while the visible quad camera subsystem was calibrated down to 0.036 pix. In the process of calibration we acquired 3 sequences of 8-frame (4 visible + 4 LWIR) image sets, 60 sets from each of the 3 camera positions: 7.4m from the target on the target center line, and 2 side views from 4.5m from the target and 2.2 m right and left from the center line. From each position camera axis was scanned in the range of ±40° horizontally and ±25° vertically.
(more…)
July 21, 2018
by Olga Filippova
In this blog article we will recall the most interesting results of Elphel participation at CVPR 2018 Expo, the conversations we had with visitor’s at the booth, FAQs as well as unusual questions, and what we learned from it. In
addition we will explain our current state of development as well as our near and far goals, and how the exhibition helps to achieve them.
The Expo lasted from June 19-21, and each day had it’s own focus and results, so this article is organized chronologically.
Day One: The best show ever!
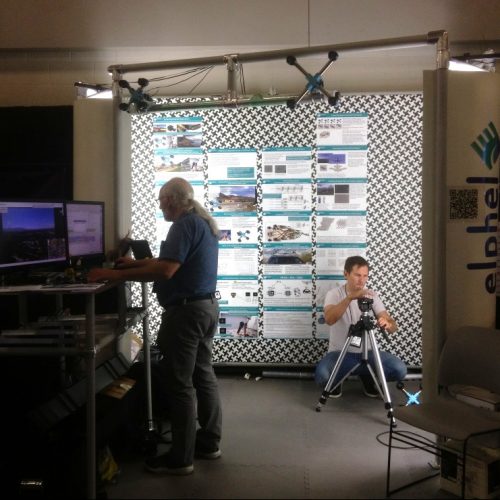
June 19, CVPR 2018, booth 132
While we are standing nervously at our booth, thinking: “Is there going to be any interest? Will people come, will they ask questions?”, the first poster session starts and a wave of visitors floods the exhibition floor. Our first guest at the booth spends 30 minutes, knowledgeably inquiring about Elphel’s long-range 3D technology and leaves his business card, saying that he is very impressed. This was a good start of a very busy day full of technical discussions. CVPR is the
first exhibition we have participated in where we did not have any problems explaining our projects.
The most common questions that were asked:
(more…)
July 20, 2018
by Andrey Filippov
We uploaded an image set with 2D correlation data together with the import Python code for experiments with the neural networks and are now looking for collaboration with those who would love to apply their DL experience to the new kind of input data. More data will follow and we welcome feedback to make this data set more useful.
The application area we are interested in is an extremely long distance 3D scene reconstruction and ranging with the distance to baseline ratio of 1000:1 to 10,000:1 while preserving wide field of view. Earlier post describes aircraft distance and velocity measurements with up to 3,000:1 distance-to-baseline ratio and 60°(H)×45°(V) field of view.
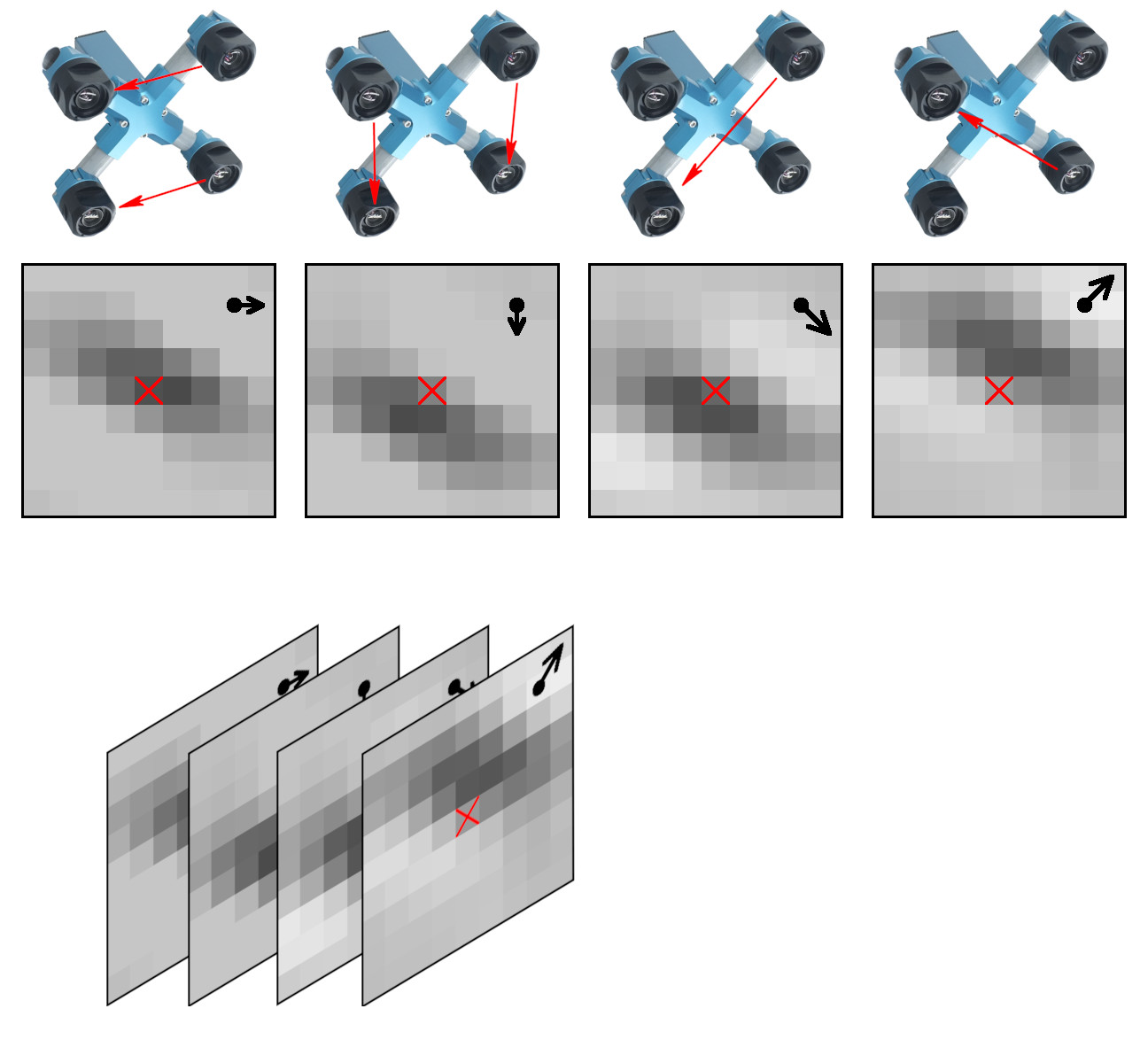
Figure 1. Space-invariant 2D phase correlation data
Data set: source images, 2D correlation tiles, and X3D scene models
The data set contains 2D phase correlation output calculated from the 2592×1936 Bayer mosaic source images captured by the quad stereo camera, and Disparity Space Image (DSI) calculated from a pair of such cameras. Longer baseline provides higher range resolution and this DSI is serving as the ground truth for a single quad camera. The source images as well as all the used software is also provided under the GNU/GPLv3 license. DSI is organized as a 324×242 array – each sample is calculated from the corresponding 16×16 tile. Tiles are overlapping (as shown in Figure 3) with stride 8.
These data sets are provided together with the fully reconstructed X3D scene models, viewable in the browser (Wavefront OBJ files are also generated). The scene models are different from the raw DSI as the next software stages generate meshes, and that frequently leads to over-simplification of the original DSI (so most fronto parallel objects in the scene provide better range accuracy when probed near the bottom). Each scene is accompanied with the multi-layer TIFF file (*-DSI_COMBO.tiff) that allows to see the difference between the measured DSI and the one used in the rendered X3D model. File format and Python import software is documented in Oleg’s post “Reading quad stereo TIFF image stacks in Python and formatting data for TensorFlow”, the data files are directly viewable with ImageJ.
(more…)
May 6, 2018
by Andrey Filippov
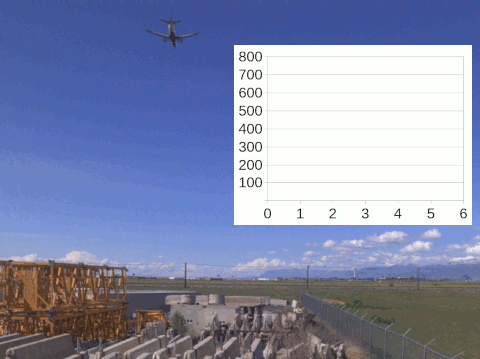
Figure 1. Aircraft positions during descent captured with the quad stereo camera. Each animation frame corresponds to the available 3-D model.
While we continue to work on the multi-sensor stereo camera hardware (we plan to double the number of sensors to capture single-exposure HDR image sets) and develop code to get the ground truth data for the CNN training, we had some fun testing the camera for capturing aircraft position in 3-D space. Completely passive method, of course.
We found a suitable spot about 2.5 km from the beginning of the runway 34L of the Salt Lake City international airport (exact location is shown in the model viewer) so approaching aircraft would pass almost over our heads. With the 60°(H)×45°(V) field of view of the camera aircraft are visible when they are 270 m away horizontally.
(more…)
February 5, 2018
by Andrey Filippov
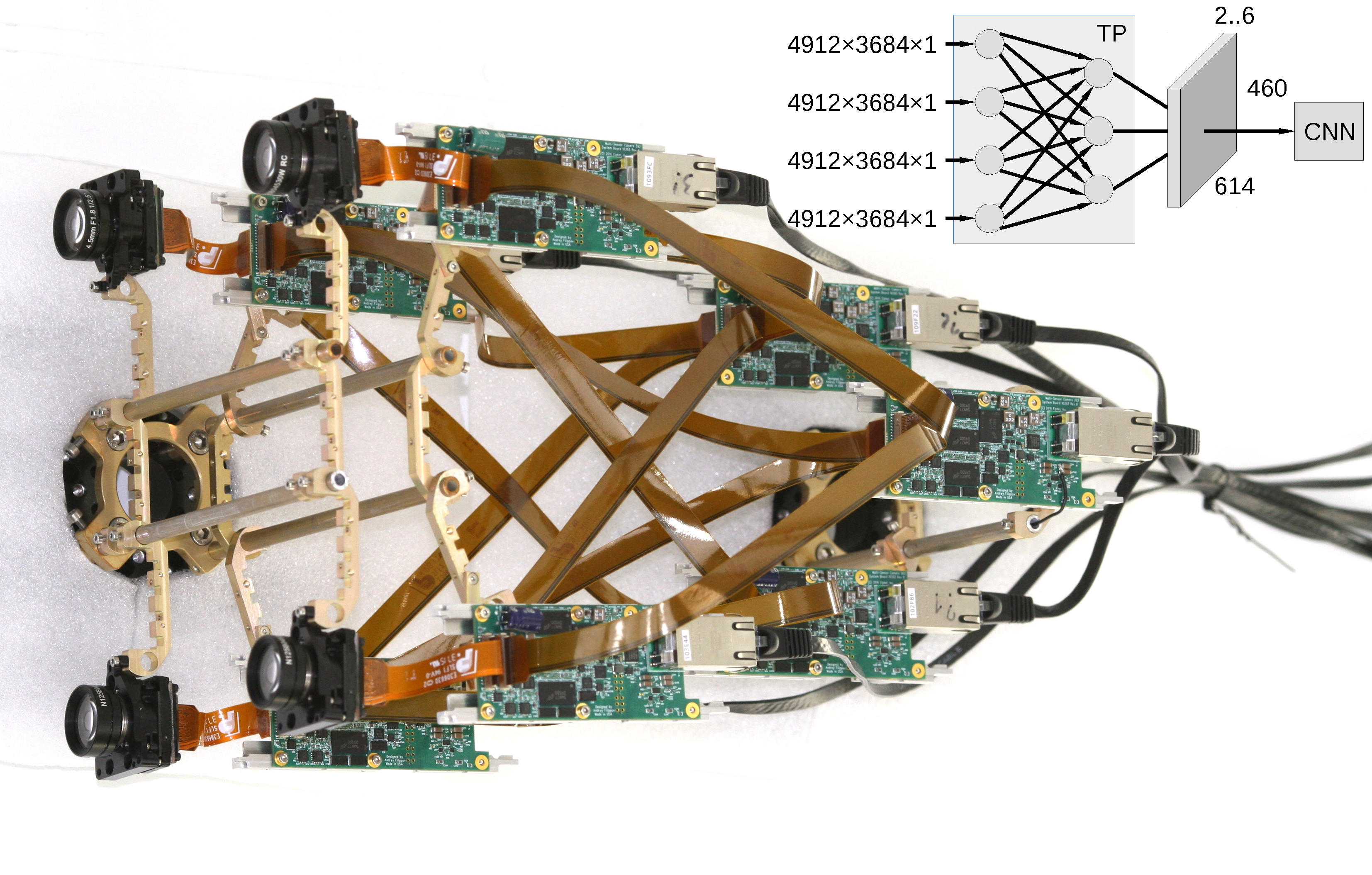
Figure 1. Multi-board setup for the TP+CNN prototype
Featured on Image Sensors World
This article describes our next steps that will continue the year-long research on high resolution multi-view stereo for long distance ranging and 3-D reconstruction. We plan to fuse the methods of high resolution images calibration and processing, already emulated functionality of the Tile Processor (TP), RTL code developed for its implementation and the Convolutional Neural Network (CNN). Compared to the CNN alone this approach promises over a hundred times reduction in the number of input features without sacrificing universality of the end-to-end processing. The TP part of the system is responsible for the high resolution aspects of the image acquisition (such as optical aberrations correction and image rectification), preserves deep sub-pixel super-resolution using efficient implementation of the 2-D linear transforms. Tile processor is free of any training, only a few hyperparameters define its operation, all the application-specific processing and “decision making” is delegated to the CNN.
(more…)
December 13, 2017
by Olga Filippova
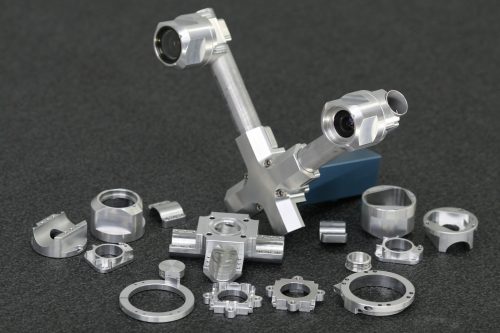
MNC393-XCAM partial assembly and parts
The long anticipated parts for the Long range camera have arrived!
The mechanical parts for the MNC393-XCAM –
Long Range Multi-view Stereo Camera are machined, tested, and ready to be anodized. This enables us to have the X-camera assembled before the winter holidays. The holiday break will provide a good opportunity to test the camera, capture new photos, and create robust 3D models from calibrated images.
The titanium X-frame of the camera ensures thermal stability required for continuous accuracy of 3D measurements. The aluminum enclosure and sealed lens filters weatherproof the system allowing for the proposed outdoor use of the camera.
We intend to assemble two cameras: one with a 150 mm distance between the sensors and another with a longer baseline. The expected accuracy for the camera with the shorter baseline is greater than 10% at a 200 meter distance. We have achieved 10% accuracy with H-camera with calibrated sensors, even though the 3D-printed parts were not thermally stable and some error was accumulated over time. It was a very pleasant surprise that the software was still able to deal with somewhat un-calibrated images and detect distances very accurately, creating impressive 3D-scenes:
Scene_viewer The second camera will have a 280 mm distance between sensors, which is determined by the longest FPC cables we can use without signal losses. It promises to double the measured distance with the same degree of accuracy, therefore an extremely long range 3D-scenes will be produced.
The Long Range Multi-View Stereo Camera with 4 sensors MNC393-XCAM is planned for release in early 2018.
September 25, 2017
by Paulina Filippova and Fyodor Filippov
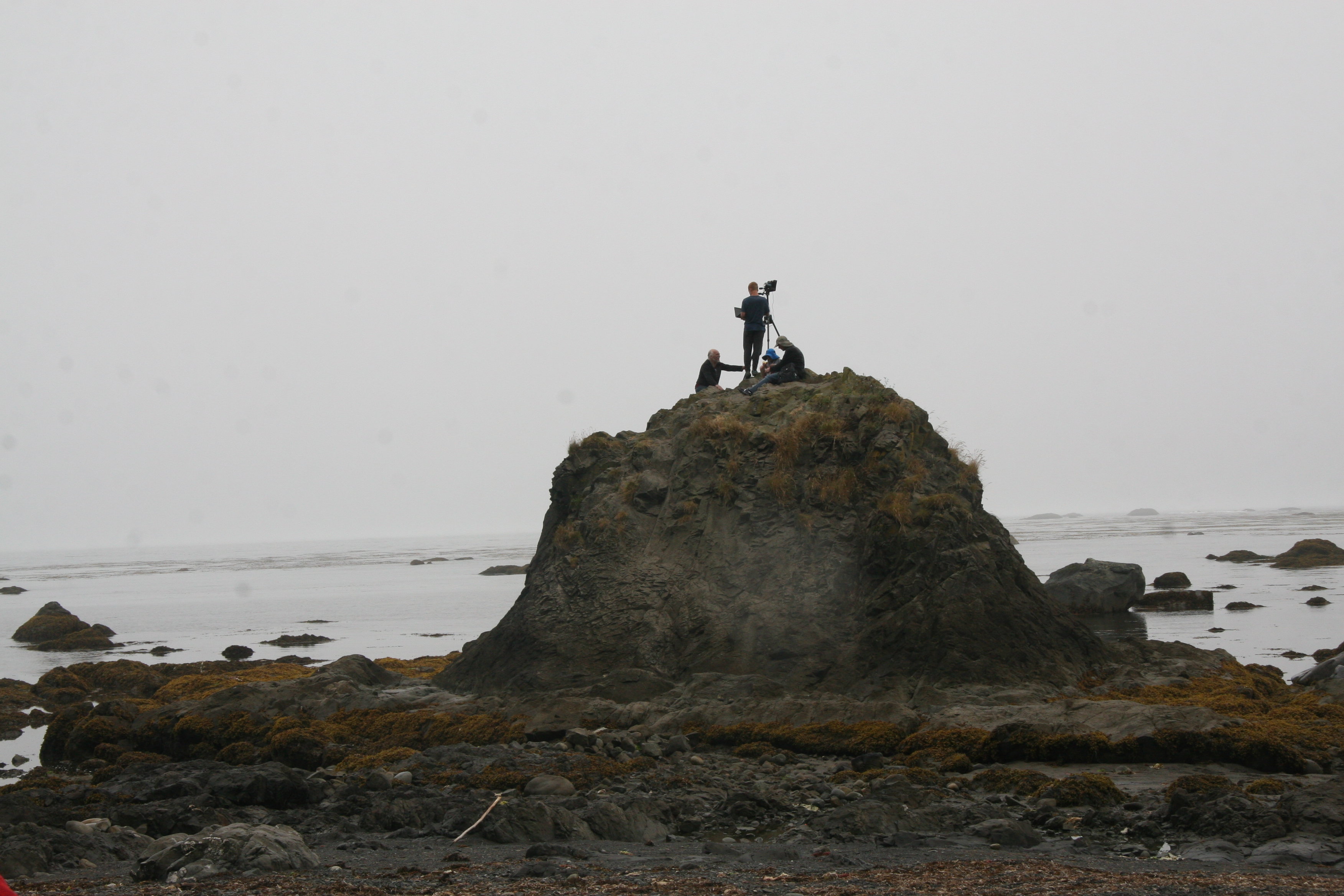
Setting 3D camera on the rock at Cape Alava
Testing 3D camera
on a road trip
In August of 2017, my family and I went on a trip to the Pacific Northwest, partially for a much needed vacation, but equally as importantly, to test my dad’s new 3D camera. My dad had been designing calibrated multi-sensor cameras for as long as I can remember, and since February was working determinately on developing principally
new algorithms for reconstructing a 3D model from a set of 4 simultaneously taken photographs . Now that the camera and the software were ready, there was no better time to test it.
(more…)
September 20, 2017
by Andrey Filippov
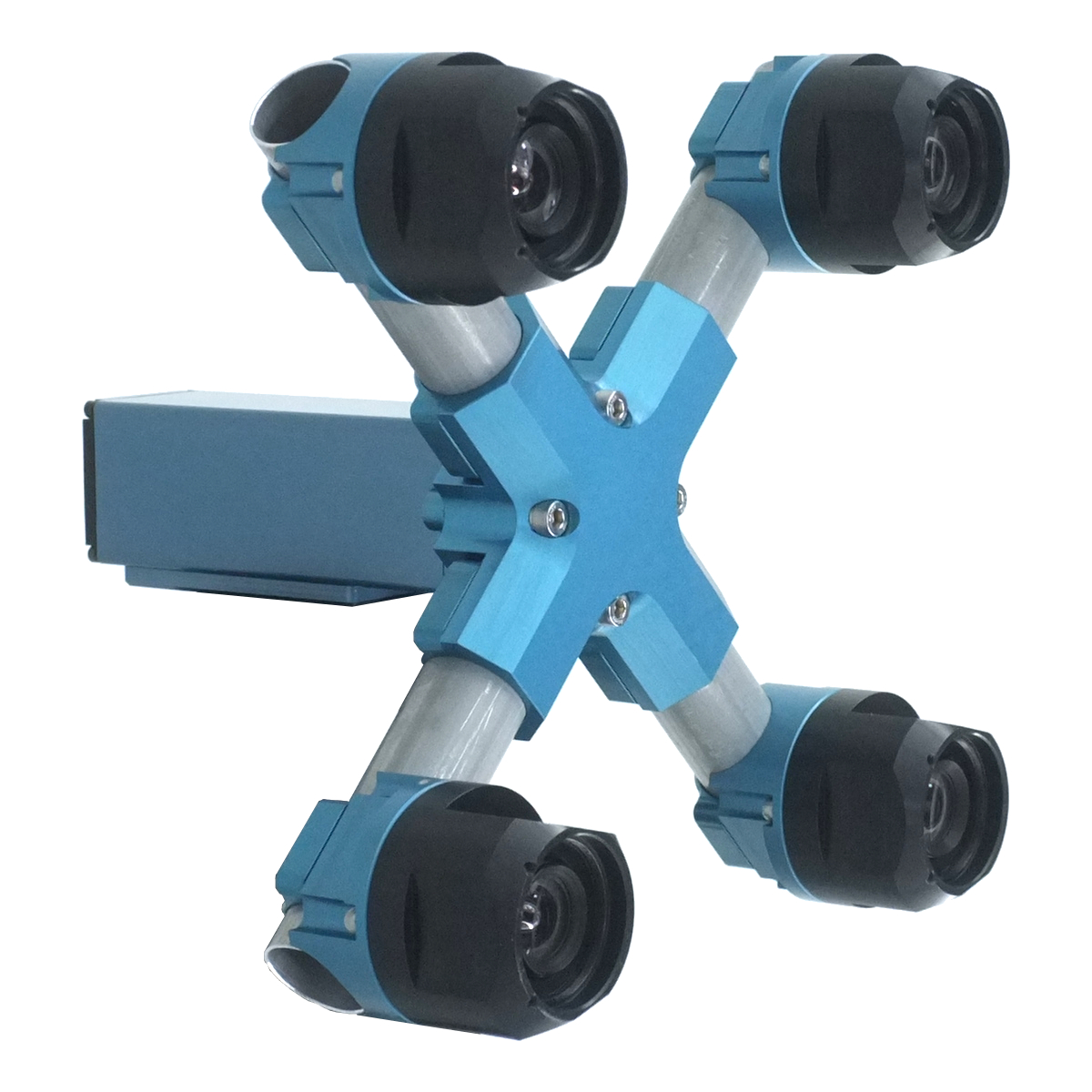
Figure 1. Four sensor stereo camera
Four-camera stereo rig prototype is capable of measuring distances thousands times exceeding the camera baseline over wide (60 by 45 degrees) field of view. With 150 mm distance between lenses it provides ranging data at 200 meters with 10% accuracy, production units will have higher accuracy. Initial implementation uses software post-processing, but the core part of the software (tile processor) is designed as FPGA simulation and will be moved to the actual FPGA of the camera for the real time applications.
Scroll down or just hyper-jump to Scene viewer for the links to see example images and reconstructed scenes.
(more…)
Next Page »